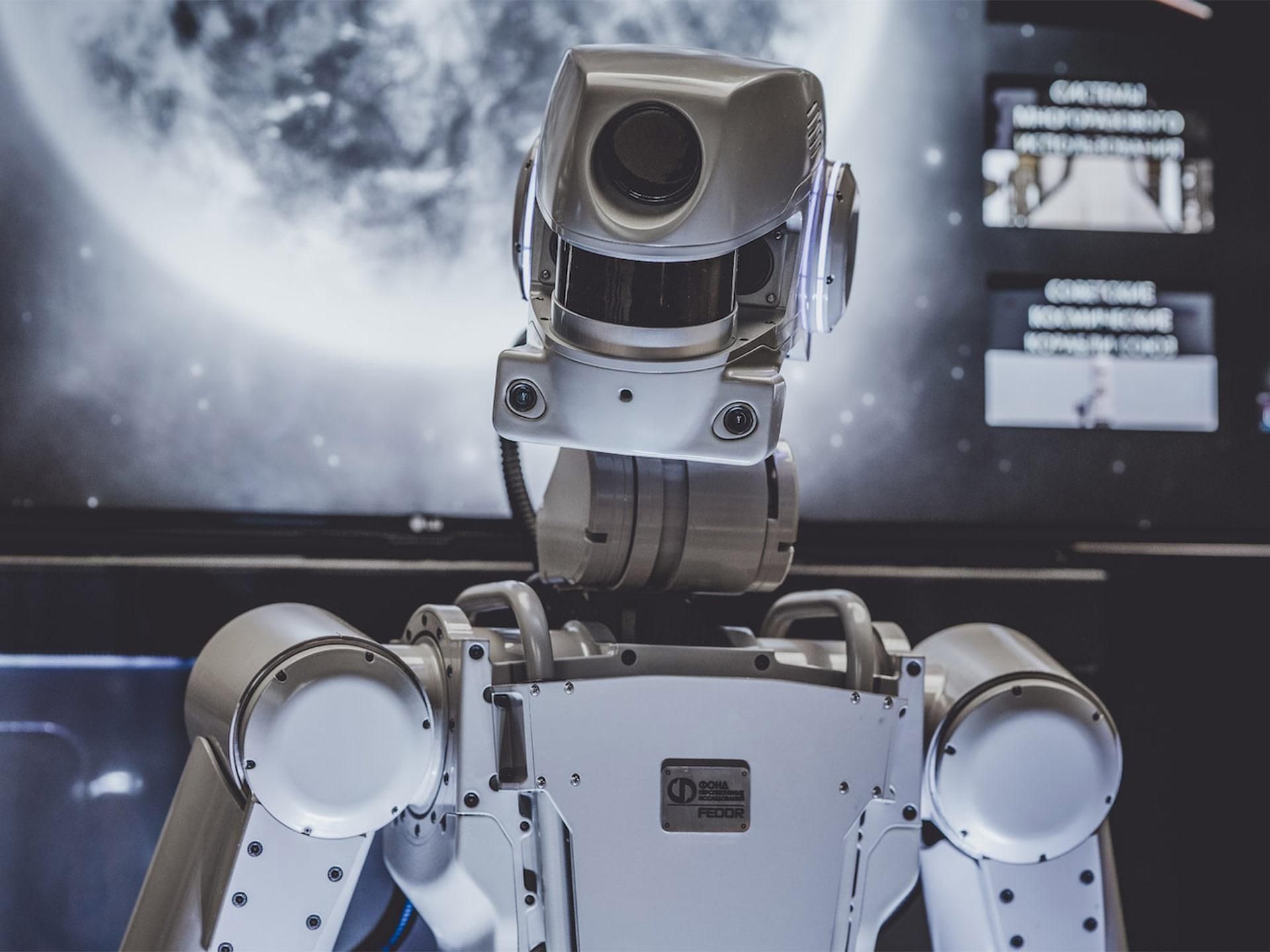
What is machine learning and where it is used
What is machine learning?
You will surely have happened to activate functions on your smartphone by saying a few simple words, or to be haunted by the advertisements of that toothbrush you looked for on Amazon the day before. Both speech recognition and tracking advertisements are part of a branch of artificial intelligence recognized as machine learning.
The term machine learning refers to all those mechanisms that allow a machine to improve its performance and functions over time through experience. Machine learning is made possible by a series of algorithms which, starting from basic notions, will be able to make a certain decision or carry out actions learned over time.
Machine learning is closely linked with computer science, mathematics, statistics, optimization and other areas of intelligent science. Its interdisciplinary nature is made up of frequent and, at times fundamental, forays into various fields.
The growth in the volume and variety of data available through increasingly sophisticated collection systems, together with cheaper and more powerful processing processes and the availability of increasingly cheap storage space, have given new life to machine learning.
Where and when machine learning is used
Machine learning is everywhere today. From online purchases to interaction with banks, from social media to predictive maintenance and quality controls, from support to diagnosis and therapies in the medical field up to data analysis and risk regulation in the stock market, with an increasingly profiled offer of content from entertainment companies to systems for creating chess and backgammon games. In general, machine learning algorithms are designed to make our experience more efficient and optimized and, especially for companies, more profitable and secure.
A bit of history
In the early 1950s, some mathematicians and statisticians began to think about applying probabilistic methods to the development of machines to enable them to make decisions based on the probability of an occurring event. As it often happens, the road to the evolution of machine learning turned out to be winding, punctuated by trial and error, experimentation and skepticism. The 1980s saw the first substantial investments in the sector, but it was only at the end of the 1990s that machine learning found a fundamental impulse in a series of innovative techniques linked to statistical and probabilistic elements.
What are the learning methods?
Machine learning uses algorithms that learn from data iteratively. Repetition is critical because the more models are exposed to the data, the more machines will be able to produce reliable results based on previous processing.
Based on the algorithms used and above all on the purposes for which the machine is built, there are 3 different modes of automatic learning: supervised, unsupervised and for reinforcement.
1. Supervised learning
it consists in providing the information system with specific and coded notions that serve as a model and allow for the construction of a database of information and experiences. When the machine has to solve a problem, it analyzes the experiences and the data entered into it and will choose the answer to give based on experiences already coded previously.
2. Unsupervised learning
in this case, the information entered in the system is not coded; this means that the machine draws on certain information without having examples of their use, nor knowledge of the expected results depending on the choice made. The machine itself is therefore called upon to catalog the available information, organize it and learn its use and, above all, the result they lead to. Over time, the machine learns for itself what the best results are for the different situations that arise.
3. Reinforcement learning
it requires the machine to be equipped with systems and tools, such as cameras, sensors, GPS, etc. able to monitor and understand the characteristics of the surrounding environment, which allow it to make the best choices for adapting to that environment. It is arguably the most complex type of machine learning and is the one behind unmanned cars.
Neural networks and machine learning
Neural networks are indispensable for machine learning. They are mathematical models composed of artificial neurons, indispensable for solving artificial intelligence problems. Their learning ability is inspired by the biological functioning of the human brain, made up of tightly interconnected nerve cells. Human neural networks are responsible for our ability to understand the environment and its changes and to provide the most appropriate adaptive responses to the need from time to time. How? Looking at things, learning to identify and recognize them and looking for relationships between them, or patterns.
Simplifying the question a lot, artificial neural networks detect patterns and connections between variables within even very large databases and at a much higher speed than human ones. In other words, these are models made up of interconnections of information.
What is the future of machine learning?
Machine learning is experiencing a rapid evolution. Its use has clear and significant advantages in many areas, even if, as often happens, it is accompanied by fears and mistrust about the extent of potentially unpredictable impacts it could have on our lives. To date, it is undeniable that machine learning provides significant support to health care, business enhancement and, in certain respects, safety. On the other hand, there are privacy problems, the gradual automation of various jobs, as well as the possible emergence of new services and new markets.